高级算法 (Fall 2022)/Balls into bins
Balls into Bins
Consider throwing
We are concerned with the following three questions regarding the balls into bins model:
- birthday problem: the probability that every bin contains at most one ball (the mapping is 1-1);
- coupon collector problem: the probability that every bin contains at least one ball (the mapping is on-to);
- occupancy problem: the maximum load of bins.
Birthday Problem
There are
Due to the pigeonhole principle, it is obvious that for
We can model this problem as a balls-into-bins problem.
: there is no bin with more than one balls (i.e. no two students share birthday).
We first analyze this by counting. There are totally
Thus the probability is given by:
Recall that
There is also a more "probabilistic" argument for the above equation. Consider again that
The first student has a birthday for sure. The probability that the second student has a different birthday from the first student is
which is the same as what we got by the counting argument.
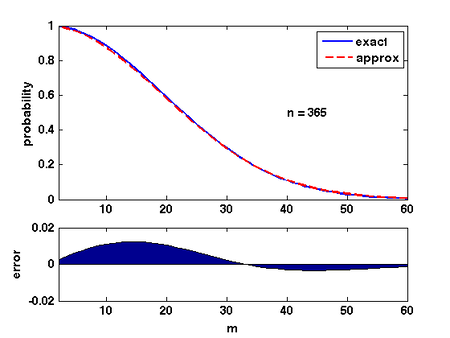
There are several ways of analyzing this formular. Here is a convenient one: Due to Taylor's expansion,
The quality of this approximation is shown in the Figure.
Therefore, for
Coupon Collector
Suppose that a chocolate company releases
The coupon collector problem can be described in the balls-into-bins model as follows. We keep throwing balls one-by-one into
Theorem - Let
be the number of balls thrown uniformly and independently to bins until no bin is empty. Then , where is the th harmonic number.
- Let
Proof. Let be the number of balls thrown while there are exactly nonempty bins, then clearly .When there are exactly
nonempty bins, throwing a ball, the probability that the number of nonempty bins increases (i.e. the ball is thrown to an empty bin) is is the number of balls thrown to make the number of nonempty bins increases from to , i.e. the number of balls thrown until a ball is thrown to a current empty bin. Thus, follows the geometric distribution, such thatFor a geometric random variable,
.Applying the linearity of expectations,
where
is the th Harmonic number, and . Thus, for the coupon collectors problem, the expected number of coupons required to obtain all types of coupons is .
Only knowing the expectation is not good enough. We would like to know how fast the probability decrease as a random variable deviates from its mean value.
Theorem - Let
be the number of balls thrown uniformly and independently to bins until no bin is empty. Then for any .
- Let
Proof. For any particular bin , the probability that bin is empty after throwing balls isBy the union bound, the probability that there exists an empty bin after throwing
balls is
Stable Marriage
We now consider the famous stable marriage problem or stable matching problem (SMP). This problem captures two aspects: allocations (matchings) and stability, two central topics in economics.
An instance of stable marriage consists of:
men and women;- each person associated with a strictly ordered preference list containing all the members of the opposite sex.
Formally, let
A matching is a one-one correspondence
Definition (stable matching) - A pair
of a man and woman is a blocking pair in a matching if and are not partners in but prefers to , and prefers to .
- A matching
is stable if there is no blocking pair in it.
- A pair
It is unclear from the definition itself whether stable matchings always exist, and how to efficiently find a stable matching. Both questions are answered by the following proposal algorithm due to Gale and Shapley.
The proposal algorithm (Gale-Shapley 1962) - Initially, all person are not married;
- in each step (called a proposal):
- an arbitrary unmarried man
proposes to the woman who is ranked highest in his preference list among all the women who has not yet rejected ; - if
is still single then accepts the proposal and is married to ; - if
is married to another man who is ranked lower than in her preference list then divorces (thus becomes single again and considers himself as rejected by ) and is married to ; - if otherwise
rejects ;
- an arbitrary unmarried man
The algorithm terminates when the last single woman receives a proposal. Since for every pair
It is obvious to see that the algorithm retruns a macthing, and this matching must be stable. To see this, by contradiction suppose that the algorithm resturns a macthing
We are interested in the average-case performance of this algorithm, that is, the expected number of proposals if everyone's preference list is a uniformly and independently random permutation.
The following principle of deferred decisions is quite useful in analysing performance of algorithm with random input.
Principle of deferred decisions - The decision of random choice in the random input can be deferred to the running time of the algorithm.
Apply the principle of deferred decisions, the deterministic proposal algorithm with random permutations as input is equivalent to the following random process:
- At each step, a man
choose a woman uniformly and independently at random to propose, among all the women who have not rejected him yet. (sample without replacement)
We then compare the above process with the following modified process:
- The man
repeatedly samples a uniform and independent woman to propose among all women, until he successfully samples a woman who has not rejected him and propose to her. (sample with replacement)
It is easy to see that the modified process (sample with replacement) is no more efficient than the original process (sample without replacement) because it simulates the original process if at each step we only count the last proposal to the woman who has not rejected the man. Such comparison of two random processes by forcing them to be related in some way is called coupling.
Note that in the modified process (sample with replacement), each proposal, no matter from which man, is going to a uniformly and independently random women. And we know that the algorithm terminated once the last single woman receives a proposal, i.e. once all
Occupancy Problem
Now we ask about the loads of bins. Assuming that
An easy analysis shows that for every bin
Because there are totally
Therefore, due to the linearity of expectations,
Because for each ball, the bin to which the ball is assigned is uniformly and independently chosen, the distributions of the loads of bins are identical. Thus
Next we analyze the distribution of the maximum load. We show that when
Theorem - Suppose that
balls are thrown independently and uniformly at random into bins. For , let be the random variable denoting the number of balls in the th bin. Then
- Suppose that
Proof. Let be an integer. Take bin 1. For any particular balls, these balls are all thrown to bin 1 with probability , and there are totally distinct sets of balls. Therefore, applying the union bound,According to Stirling's approximation,
, thusFigure 1 Due to the symmetry. All
have the same distribution. Apply the union bound again,When
,Therefore,
When
Formally, it can be proved that for
Universal Hashing
Hashing is one of the oldest tools in Computer Science. Knuth's memorandum in 1963 on analysis of hash tables is now considered to be the birth of the area of analysis of algorithms.
- Knuth. Notes on "open" addressing, July 22 1963. Unpublished memorandum.
The idea of hashing is simple: an unknown set
This idea seems clever: we use a consistent mapping to deal with an arbitrary unknown data set. However, there is a fundamental flaw for hashing.
- For sufficiently large universe (
), for any function, there exists a bad data set , such that all items in are mapped to the same entry in the table.
A simple use of pigeonhole principle can prove the above statement.
To overcome this situation, randomization is introduced into hashing. We assume that the hash function is a random mapping from
Simple Uniform Hash Assumption (SUHA or UHA, a.k.a. the random oracle model):
- A uniform random function
is available and the computation of is efficient.
Families of universal hash functions
The assumption of completely random function simplifies the analysis. However, in practice, truly uniform random hash function is extremely expensive to compute and store. Thus, this simple assumption can hardly represent the reality.
There are two approaches for implementing practical hash functions. One is to use ad hoc implementations and wish they may work. The other approach is to construct class of hash functions which are efficient to compute and store but with weaker randomness guarantees, and then analyze the applications of hash functions based on this weaker assumption of randomness.
This route was took by Carter and Wegman in 1977 while they introduced universal families of hash functions.
Definition (universal hash families) - Let
be a universe with . A family of hash functions from to is said to be -universal if, for any items and for a hash function chosen uniformly at random from , we have
- A family of hash functions
from to is said to be strongly -universal if, for any items , any values , and for a hash function chosen uniformly at random from , we have
- Let
In particular, for a 2-universal family
For a strongly 2-universal family
This behavior is exactly the same as uniform random hash functions on any pair of inputs. For this reason, a strongly 2-universal hash family are also called pairwise independent hash functions.
2-universal hash families
The construction of pairwise independent random variables via modulo a prime introduced in Section 1 already provides a way of constructing a strongly 2-universal hash family.
Let
and the family is
Lemma is strongly 2-universal.
Proof. In Section 1, we have proved the pairwise independence of the sequence of , for , which directly implies that is strongly 2-universal.
- The original construction of Carter-Wegman
What if we want to have hash functions from
Suppose that the universe is
and the family
Note that unlike the first construction, now
Lemma (Carter-Wegman) is 2-universal.
Proof. Due to the definition of , there are many different hash functions in , because each hash function in corresponds to a pair of and . We only need to count for any particular pair of that , the number of hash functions that .We first note that for any
, . This is because would imply that , which can never happen since and (note that for an ). Therefore, we can assume that and for .By linear algebra (over finite field), for any
that , for any that , there is exact one solution to satisfying:After modulo
, every has at most many that but . Therefore, for every pair of that , there exist at most pairs of and such that , which means there are at most many hash functions having for . For uniformly chosen from , for any ,We prove that
is 2-universal.
- A construction used in practice
The main issue of Carter-Wegman construction is the efficiency. The mod operation is very slow, and has been so for more than 30 years.
The following construction is due to Dietzfelbinger et al. It was published in 1997 and has been practically used in various applications of universal hashing.
The family of hash functions is from
and the family
This family of hash functions does not exactly meet the requirement of 2-universal family. However, Dietzfelbinger et al proved that
So
The function is extremely simple to compute in c language.
We exploit that C-multiplication (*) of unsigned u-bit numbers is done
h_a(x) = (a*x)>>(u-v)
The bit-wise shifting is a lot faster than modular. It explains the popularity of this scheme in practice than the original Carter-Wegman construction.
Collision number
Consider a 2-universal family
As in the balls-into-bins with full independence, we are curious about the questions such as the birthday problem or the maximum load. These questions are interesting not only because they are natural to ask in a balls-into-bins setting, but in the context of hashing, they are closely related to the performance of hash functions.
The old techniques for analyzing balls-into-bins rely too much on the independence of the choice of the bin for each ball, therefore can hardly be extended to the setting of 2-universal hash families. However, it turns out several balls-into-bins questions can somehow be answered by analyzing a very natural quantity: the number of collision pairs.
A collision pair for hashing is a pair of elements
The total number of collision pairs among the
Since
The expected number of collision pairs is
In particular, for
Birthday problem
In the context of hash functions, the birthday problem ask for the probability that there is no collision at all. Since collision is something that we want to avoid in the applications of hash functions, we would like to lower bound the probability of zero-collision, i.e. to upper bound the probability that there exists a collision pair.
The above analysis gives us an estimation on the expected number of collision pairs, such that
When
Theorem - If
is chosen uniformly from a 2-universal family of hash functions mapping the universe to where , then for any set of items, where , the probability that there exits a collision pair is
- If
Recall that for mutually independent choices of bins, for some